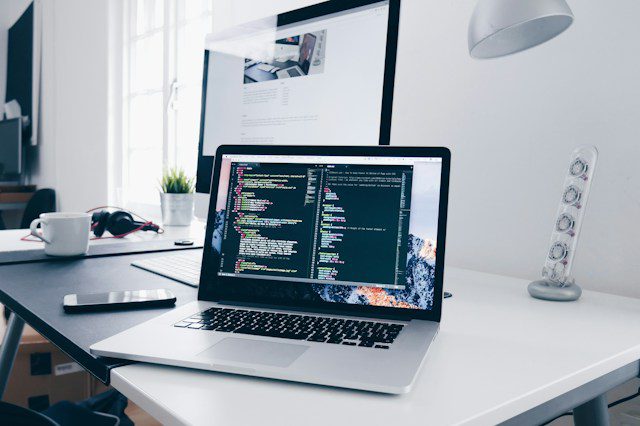
In the contemporary data-driven world, data science has emerged as a pivotal force driving innovation, decision-making, and problem-solving across various industries. From identifying trends to making predictions, data scientists leverage a systematic approach known as the Data Science Lifecycle to extract actionable insights from raw data. In this blog, we’ll delve into the intricacies of the Data Science Lifecycle, exploring each stage in detail and elucidating how this structured framework enables organizations to harness the power of data effectively. Whether you’re a seasoned data professional or an enthusiast, join us as we uncover the mysteries behind one of the most transformative processes in modern analytics.
Data science presents a vast scope as a career option in India, with burgeoning opportunities across industries like IT, finance, healthcare, and e-commerce. The demand for skilled and qualified data scientist professionals continues to soar as businesses seek to extract valuable insights from the given data to drive decision-making and innovation. Pursuing a data science course in Bangalore can offer a strategic advantage, given the city’s status as a hub for technology and innovation. By gaining expertise in cutting-edge tools and techniques, such as machine learning and big data analytics, aspiring data scientists can stay ahead of the curve and capitalize on the abundance of career prospects in this dynamic field.
Data science is a multifaceted field that involves extracting insights and knowledge from structured and unstructured data. It encompasses various techniques, including statistical analysis, machine learning, data mining, and visualization, to uncover patterns, trends, and correlations within datasets. The Data Science Lifecycle is the systematic process of approaching data-driven projects, typically comprising data collection, preparation, exploratory data analysis, model building, model deployment, and monitoring. Each stage of the lifecycle is crucial for ensuring the accuracy, relevance, and effectiveness of the insights derived from data. By following this structured framework, data scientists can streamline their workflow, optimize resource allocation, and ultimately, drive informed decision-making within organizations.
The Data Science Lifecycle
Data Acquisition: Data acquisition marks the inception of any data-driven project, involving the systematic collection of raw data from diverse sources including databases, APIs, files, or even sensors. It’s imperative to ensure that the data collected is relevant to the problem statement at hand and adheres to legal and ethical considerations. This phase often requires understanding the data schema, access permissions, and potential quality issues. Data scientists employ various techniques to retrieve data, including web scraping for online sources or utilizing data pipelines for streaming data. Ultimately, the goal is to gather a comprehensive dataset that serves as the foundation for subsequent analysis and modeling efforts.
Data Preparation: Once the raw data is acquired, it undergoes a series of preprocessing steps in the data preparation phase to make it suitable for analysis. This involves cleaning the data to handle missing values, removing duplicates, and correcting errors. Additionally, data may be transformed through techniques like normalization, standardization, or encoding to ensure consistency and comparability across different features. Feature engineering also takes place during this phase, where new features are created or existing ones are transformed to extract more meaningful insights. Data preparation lays the groundwork for accurate analysis and modeling by ensuring that the data is clean, structured, and ready for further exploration.
Exploratory Data Analysis (EDA): Exploratory Data Analysis (EDA) is a critical phase where data scientists explore and analyze the prepared dataset to uncover patterns, trends, and relationships. They gain insights into the data’s characteristics and distributions through various statistical methods and data visualization techniques such as histograms, scatter plots, and heatmaps. EDA helps understand outliers, detect anomalies, and identify potential correlations between variables. By thoroughly exploring the data, data scientists can formulate hypotheses, validate assumptions, and guide the subsequent modeling process effectively.
Model Development: Model development is where machine learning models are constructed and trained using the analyzed data. Data is typically divided into training, validation, and test sets to develop and evaluate models effectively. Data scientists select appropriate algorithms based on the nature of the problem, whether it’s regression, classification, clustering, or deep learning. They fine-tune model hyperparameters and assess performance using metrics such as accuracy, precision, recall, or F1-score. Model development aims to create predictive or descriptive models that accurately capture underlying patterns in the data and generalize well to unseen instances.
Model Deployment: Following successful model development and evaluation, the next step is model deployment, where the trained model is integrated into a production environment to make real-time predictions on new data. This phase involves deploying the model through various channels such as cloud services, APIs, or embedded systems, depending on the application’s requirements. Integration with existing systems or applications is crucial to automate decision-making processes and drive business value. Continuous monitoring of the deployed model’s performance ensures its reliability and effectiveness in generating accurate predictions in real-world scenarios.
Model Maintenance and Monitoring: Model maintenance and monitoring are ongoing processes to ensure the deployed model’s reliability, performance, and relevance over time. This involves monitoring key performance metrics, detecting data drift or concept drift, and updating the model to adapt to changing circumstances. Regular retraining of the model with new data helps maintain its accuracy and generalizability. Additionally, addressing issues like software updates, scalability, security, and regulation compliance ensures the model’s robustness and compliance with industry standards. Model maintenance and monitoring are essential for maximizing the model’s utility and longevity in delivering actionable insights and driving informed decision-making.
Conclusion
Understanding the Data Science Lifecycle is crucial for navigating the complexities of data-driven projects effectively. Each stage, from data acquisition to model maintenance, plays a vital role in extracting actionable insights and driving informed decision-making. Pursuing a data science course in Bangalore can serve as a valuable stepping stone towards building a rewarding career in the data science industry. With Bangalore’s thriving tech ecosystem and renowned educational institutions, aspiring data scientists can gain hands-on experience, learn cutting-edge techniques, and network with industry professionals, equipping themselves with the skills and knowledge needed to excel in this dynamic and rapidly evolving field.